Picture 1 of 1

Picture 1 of 1
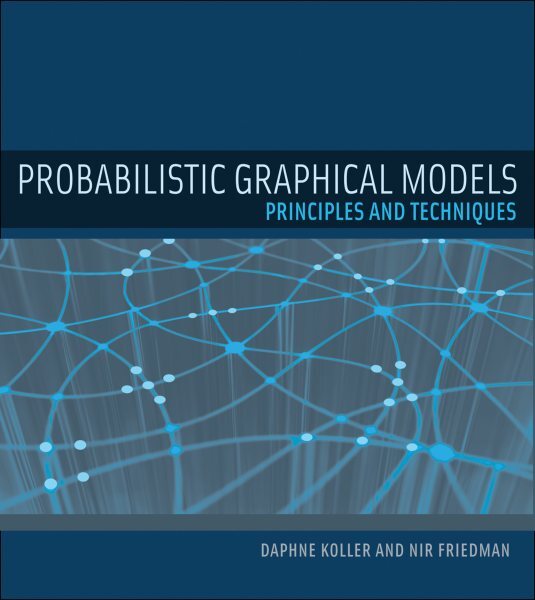
Probabilistic Graphical Models : Principles and Techniques, Hardcover by Koll...
US $138.50
ApproximatelyRM 582.39
Condition:
Brand New
A new, unread, unused book in perfect condition with no missing or damaged pages.
2 available
Postage:
Free Economy Shipping.
Located in: Jessup, Maryland, United States
Delivery:
Estimated between Tue, 1 Oct and Sat, 5 Oct to 43230
Returns:
14 days return. Buyer pays for return shipping.
Coverage:
Read item description or contact seller for details. See all detailsSee all details on coverage
(Not eligible for eBay purchase protection programmes)
Seller assumes all responsibility for this listing.
eBay item number:364832963328
Item specifics
- Condition
- Brand New: A new, unread, unused book in perfect condition with no missing or damaged pages. See all condition definitionsopens in a new window or tab
- Book Title
- Probabilistic Graphical Models : Principles and Techniques
- ISBN
- 9780262013192
- Subject Area
- Mathematics, Computers
- Publication Name
- Probabilistic Graphical Models : Principles and Techniques
- Publisher
- MIT Press
- Item Length
- 9.4 in
- Subject
- Programming / Algorithms, Intelligence (Ai) & Semantics, Probability & Statistics / Bayesian Analysis
- Publication Year
- 2009
- Series
- Adaptive Computation and Machine Learning Ser.
- Type
- Textbook
- Format
- Hardcover
- Language
- English
- Item Height
- 2 in
- Item Weight
- 78 Oz
- Item Width
- 8.3 in
- Number of Pages
- 1270 Pages
About this product
Product Identifiers
Publisher
MIT Press
ISBN-10
0262013193
ISBN-13
9780262013192
eBay Product ID (ePID)
73169822
Product Key Features
Number of Pages
1270 Pages
Language
English
Publication Name
Probabilistic Graphical Models : Principles and Techniques
Publication Year
2009
Subject
Programming / Algorithms, Intelligence (Ai) & Semantics, Probability & Statistics / Bayesian Analysis
Type
Textbook
Subject Area
Mathematics, Computers
Series
Adaptive Computation and Machine Learning Ser.
Format
Hardcover
Dimensions
Item Height
2 in
Item Weight
78 Oz
Item Length
9.4 in
Item Width
8.3 in
Additional Product Features
Intended Audience
Trade
LCCN
2009-008615
Reviews
"This landmark book provides a very extensive coverage of the field, ranging from basic representational issues to the latest techniques for approximate inference and learning. As such, it is likely to become a definitive reference for all those who work in this area. Detailed worked examples and case studies also make the book accessible to students." -Kevin Murphy, Department of Computer Science, University of British Columbia
Dewey Edition
22
Illustrated
Yes
Dewey Decimal
519.5/420285
Synopsis
A general framework for constructing and using probabilistic models of complex systems that would enable a computer to use available information for making decisions., A general framework for constructing and using probabilistic models of complex systems that would enable a computer to use available information for making decisions. Most tasks require a person or an automated system to reason-to reach conclusions based on available information. The framework of probabilistic graphical models, presented in this book, provides a general approach for this task. The approach is model-based, allowing interpretable models to be constructed and then manipulated by reasoning algorithms. These models can also be learned automatically from data, allowing the approach to be used in cases where manually constructing a model is difficult or even impossible. Because uncertainty is an inescapable aspect of most real-world applications, the book focuses on probabilistic models, which make the uncertainty explicit and provide models that are more faithful to reality. Probabilistic Graphical Models discusses a variety of models, spanning Bayesian networks, undirected Markov networks, discrete and continuous models, and extensions to deal with dynamical systems and relational data. For each class of models, the text describes the three fundamental cornerstones- representation, inference, and learning, presenting both basic concepts and advanced techniques. Finally, the book considers the use of the proposed framework for causal reasoning and decision making under uncertainty. The main text in each chapter provides the detailed technical development of the key ideas. Most chapters also include boxes with additional material- skill boxes, which describe techniques; case study boxes, which discuss empirical cases related to the approach described in the text, including applications in computer vision, robotics, natural language understanding, and computational biology; and concept boxes, which present significant concepts drawn from the material in the chapter. Instructors (and readers) can group chapters in various combinations, from core topics to more technically advanced material, to suit their particular needs., A general framework for constructing and using probabilistic models of complex systems that would enable a computer to use available information for making decisions. Most tasks require a person or an automated system to reason--to reach conclusions based on available information. The framework of probabilistic graphical models, presented in this book, provides a general approach for this task. The approach is model-based, allowing interpretable models to be constructed and then manipulated by reasoning algorithms. These models can also be learned automatically from data, allowing the approach to be used in cases where manually constructing a model is difficult or even impossible. Because uncertainty is an inescapable aspect of most real-world applications, the book focuses on probabilistic models, which make the uncertainty explicit and provide models that are more faithful to reality. Probabilistic Graphical Models discusses a variety of models, spanning Bayesian networks, undirected Markov networks, discrete and continuous models, and extensions to deal with dynamical systems and relational data. For each class of models, the text describes the three fundamental cornerstones: representation, inference, and learning, presenting both basic concepts and advanced techniques. Finally, the book considers the use of the proposed framework for causal reasoning and decision making under uncertainty. The main text in each chapter provides the detailed technical development of the key ideas. Most chapters also include boxes with additional material: skill boxes, which describe techniques; case study boxes, which discuss empirical cases related to the approach described in the text, including applications in computer vision, robotics, natural language understanding, and computational biology; and concept boxes, which present significant concepts drawn from the material in the chapter. Instructors (and readers) can group chapters in various combinations, from core topics to more technically advanced material, to suit their particular needs.
LC Classification Number
QA279.5.K65 2010
Item description from the seller
Seller feedback (353,411)
- s***a (347)- Feedback left by buyer.Past monthVerified purchaseGreat
- r***e (85)- Feedback left by buyer.Past monthVerified purchaseFast shipping, great product +++
- s***l (416)- Feedback left by buyer.Past monthVerified purchaseExcellent transaction
More to explore :
- Photography Techniques Hardcover Nonfiction Books,
- Management Techniques Hardcover Nonfiction Books,
- Photography Techniques Hardcover Nonfiction Books Illustrated,
- Art & Culture Hardcover Nonfiction Photography Techniques Books,
- Nonfiction Photography Techniques Hardcover Books 1950-1999 Publication Year,
- Hardcovers,
- Hardcover Books,
- Graphic Design Hardcover Nonfiction Books in Japanese,
- Graphic Design Hardcover Nonfiction Books in Russian,
- Hardcover Textbooks